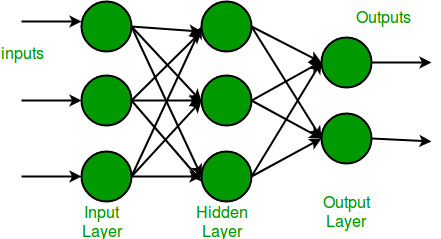
MLOps can be described as a combination of machine learning and continuous software development (DevOps). It is the continuous operation of machine learning applications. These are key to a successful ML deployment. The use of machine learning in automated machine-learning applications production is a great way of improving the accuracy and quality your software. How to set up and manage ML operations to achieve the best results
Machine learning operations
Enterprises are increasingly turning to technologies such as Deep Learning, Artificial Intelligence, and Machine Learning (ML) to automate processes and improve decision-making. To stay ahead of your competition, your company must embrace MLOps in order to maximize its impact. Machine learning can be used to improve decision making and streamline supply chain and production processes. To make this work, you must first understand MLOps in order to develop the right strategies and then implement them.
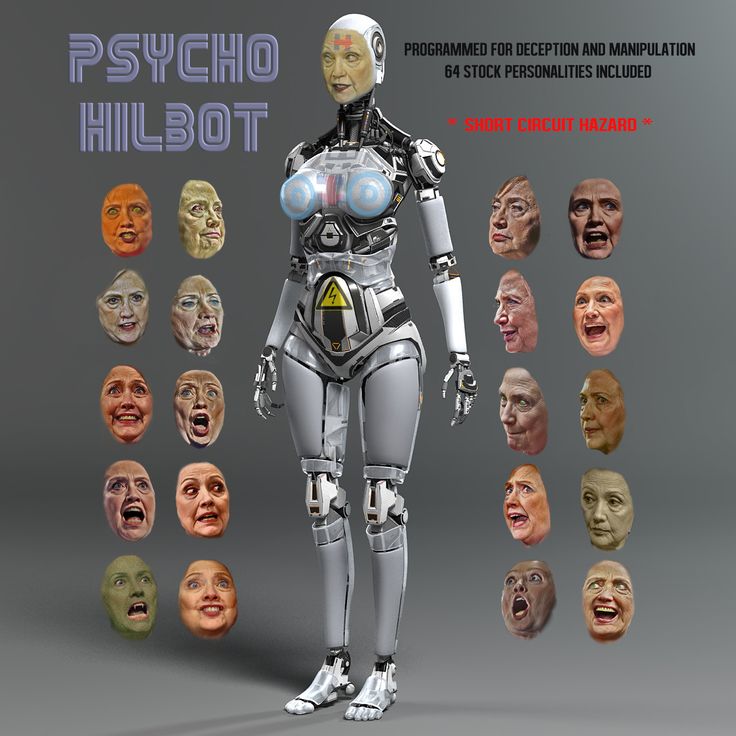
Model deployment
ML Operations are a set process to deploy and maintain Machine Learning model (ML) in production environments. Most ML models remain in the proof-of-concept stage after they are trained and deployed, and they soon become stale due to changes in the source data. This requires the rebuilding of the model as well as tracking model performance and hyperparameters. For optimal ML results, model operations is necessary.
Model monitoring
Model monitoring can be an essential component of machine-learning in operations. It is used to verify that models are working correctly and debug any issues. A live data stream is the best way to track performance changes. Then, you can create customized notifications to alert you of significant changes. This allows you to resolve any problem more quickly and effectively. Here are some tips for setting up and maintaining model monitoring in your business.
Configuration of ML models
The first step in deploying a machine learning (ML) model is to train it. The next step involves deploying it to production. This requires a variety of components, including Continuous Initiation and Continuous Delivery. The pipeline can perform continuous testing. It can also include metadata management or automated data validation. This is an essential step towards ensuring a high quality model. In the ML pipeline deployment process, configuration is often forgotten.
Validation of data
Validating ML models is an essential part of the ML process. A model that is trained with training data should be able to produce predictions that are comparable to real-life data. It is important to compare the training data with the production data in order for the model to correctly predict a particular feature. This will allow the model to be tested before being placed in a production environment. There are several steps involved in data validation.
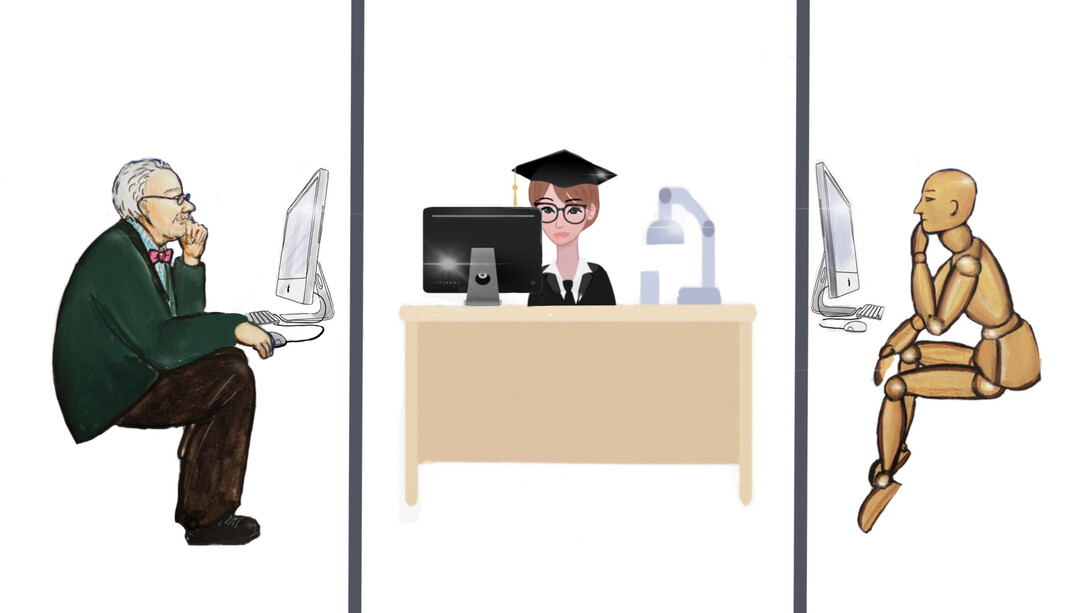
Management of change
Change management strategies are required for MLOps implementation. You need to take into account many aspects, such as your organization's maturity levels and existing processes. MLOps is possible if you are focused on just a few areas. MLOps are a great way to start your journey. One example is model reproducibility. True reproducibility is only possible through the careful implementation of source control management and model portability. For data scientists, it is possible to implement source control management processes within organizations.
FAQ
Where did AI come from?
Artificial intelligence was created in 1950 by Alan Turing, who suggested a test for intelligent machines. He stated that intelligent machines could trick people into believing they are talking to another person.
John McCarthy later took up the idea and wrote an essay titled "Can Machines Think?" in 1956. It was published in 1956.
Is Alexa an Ai?
The answer is yes. But not quite yet.
Alexa is a cloud-based voice service developed by Amazon. It allows users interact with devices by speaking.
First, the Echo smart speaker released Alexa technology. Other companies have since used similar technologies to create their own versions.
These include Google Home and Microsoft's Cortana.
Who created AI?
Alan Turing
Turing was created in 1912. His father was clergyman and his mom was a nurse. At school, he excelled at mathematics but became depressed after being rejected by Cambridge University. He took up chess and won several tournaments. He worked as a codebreaker in Britain's Bletchley Park, where he cracked German codes.
He died on April 5, 1954.
John McCarthy
McCarthy was born in 1928. He was a Princeton University mathematician before joining MIT. The LISP programming language was developed there. He had laid the foundations to modern AI by 1957.
He died in 2011.
Statistics
- In 2019, AI adoption among large companies increased by 47% compared to 2018, according to the latest Artificial IntelligenceIndex report. (marsner.com)
- More than 70 percent of users claim they book trips on their phones, review travel tips, and research local landmarks and restaurants. (builtin.com)
- By using BrainBox AI, commercial buildings can reduce total energy costs by 25% and improves occupant comfort by 60%. (analyticsinsight.net)
- Additionally, keeping in mind the current crisis, the AI is designed in a manner where it reduces the carbon footprint by 20-40%. (analyticsinsight.net)
- In the first half of 2017, the company discovered and banned 300,000 terrorist-linked accounts, 95 percent of which were found by non-human, artificially intelligent machines. (builtin.com)
External Links
How To
How do I start using AI?
Artificial intelligence can be used to create algorithms that learn from their mistakes. The algorithm can then be improved upon by applying this learning.
You could, for example, add a feature that suggests words to complete your sentence if you are writing a text message. It would use past messages to recommend similar phrases so you can choose.
You'd have to train the system first, though, to make sure it knows what you mean when you ask it to write something.
You can even create a chatbot to respond to your questions. So, for example, you might want to know "What time is my flight?" The bot will tell you that the next flight leaves at 8 a.m.
If you want to know how to get started with machine learning, take a look at our guide.